UC AI Congress participants met in seven breakout groups to discuss AI topics related to their work and interests. Each group submitted write ups about their key takeaways and goals for the UC system. Breakout session topics included:
- AI and Climate / Ag Tech, led by Josh Viers, UC Merced, AgAID UC Merced, AI Institute for Transforming Workforce and Decision Support (AgAID)
- AI for National Security and Cybersecurity, led by Brian Spears, Lawrence Livermore National Lab
- AI for Health Care, led by Alpesh Amin, UC Irvine
- AI for Teaching and Learning, led by Tamara Tate, UC Irvine
- AI and the Creative Sector, led by Jeff Burke, UCLA
- AI in Computational Research Applications and Research Integrity, led by Ilkay Altintas, UC San Diego
- Ethical Review and Human Subjects Research Involving AI, led by Ida Sim, UCSF
Breakout 1 – AI and Climate/Ag Tech – key takeaways
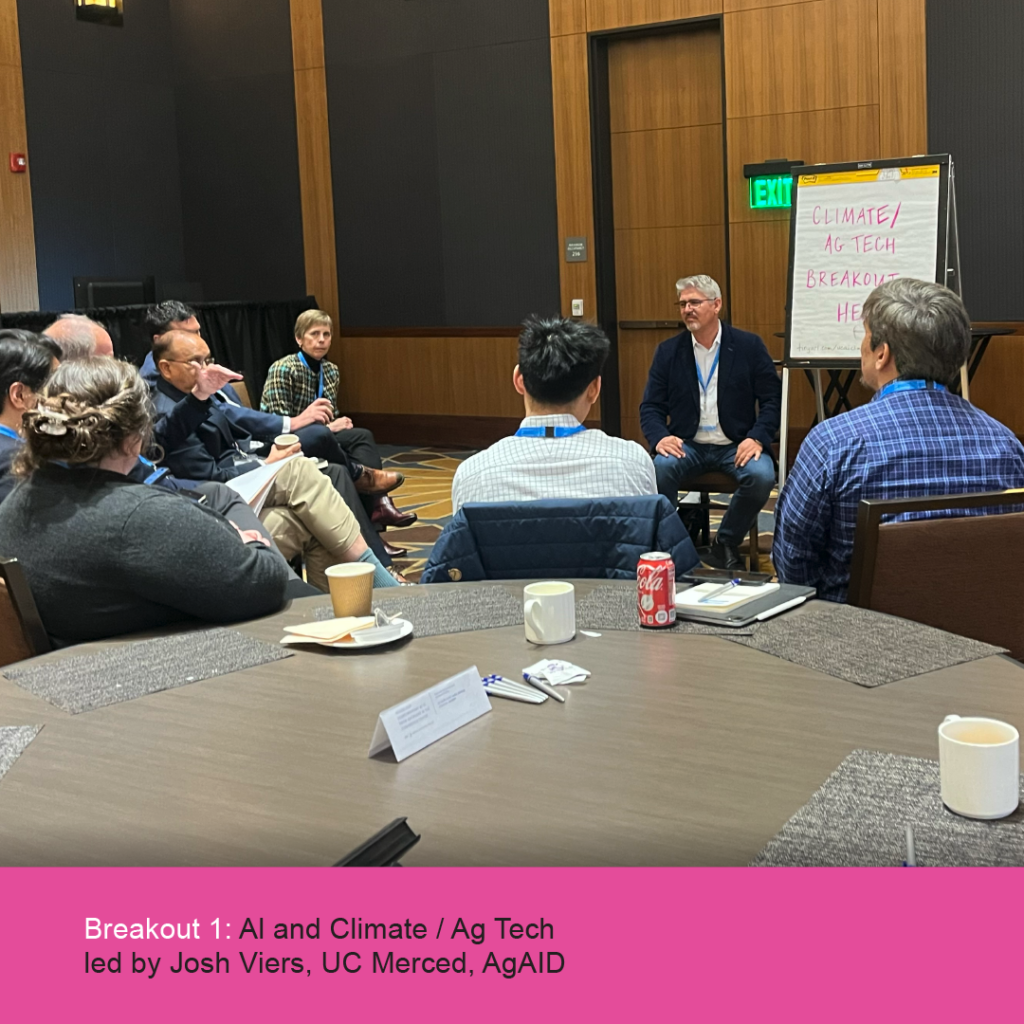
1. Leverage AI to become more proactive than reactive in AgTech
- Predict invasion of pests, fungi, etc. and genetically enhanced crops to be resilient to these threats.
- Predict land fallowing and broader impact on communities
2. Increase dispersion of knowledge. Every county could have a tech translator who can make information available for the general public.
3. UC GPT to address data quality
- Ground our own models to use our own institutional knowledge by deploying a UC GPT or Research-assisted generation (RAG) tool.
- Note that this is time consuming work, and not incentivized or compensated. Could create a requirement for PIs to share that data. Perhaps replicate a center within ANR something like the Center for Data Driven Insights for UC Health.
Breakout 2 – AI for National Security and Cybersecurity – key takeaways
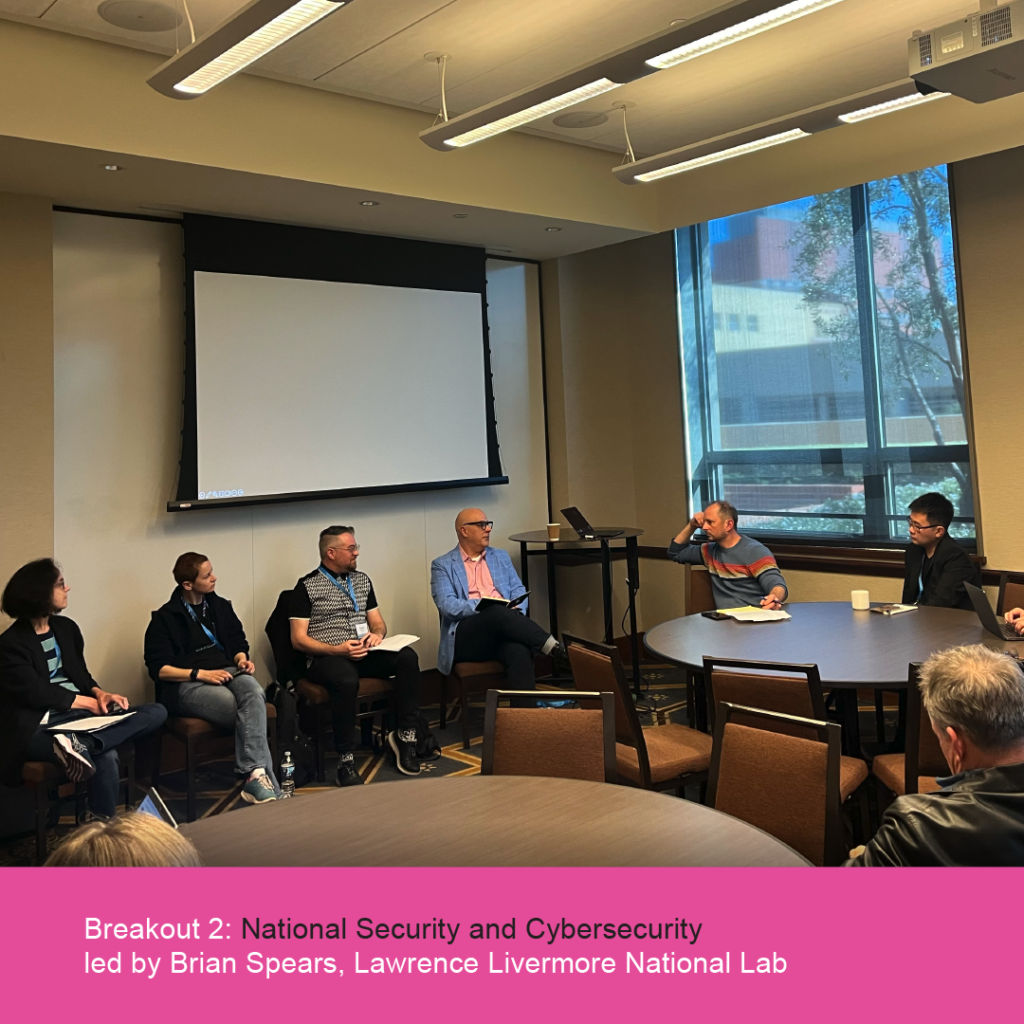
UC needs to invest in:
- Transformational infrastructure in response to AI to have world-leading operations and research.
- World-leading federated compute resource – tens of thousands of GPUs
- Capability for executing quality control on UC’s models and data
- Policies to standardize and guide risk assessment and tolerance
- A council to guide it with a strong organizational structure that can keep up with the pace needed
Breakout 3 – AI for Healthcare – key takeaways
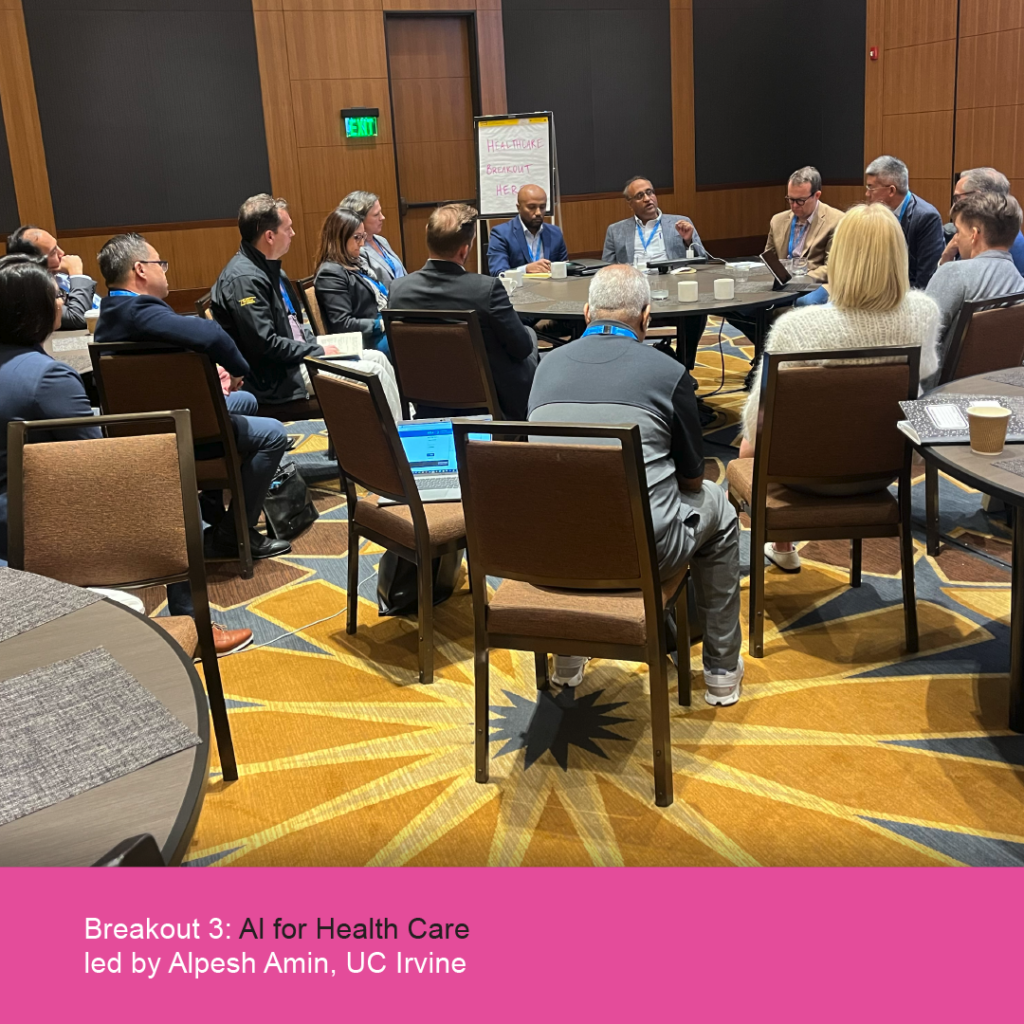
Integration and Potential Benefits: AI is being actively integrated into healthcare by medical professionals to improve the quality of care, reduce costs, and enhance patient and physician experiences. Current use cases at UC include the use of AI to simplify clinical workflows and the implementation of AI scribes to minimize administrative burdens on healthcare providers.
Challenges and Concerns: While AI offers numerous advantages, there are significant challenges such as potential biases in algorithms, privacy risks, and the over-reliance on AI for medical diagnoses. Healthcare professionals express concerns about the need for human judgment in conjunction with AI to ensure balanced and effective patient care.
Future Directions and Governance: Discussions highlight the need for robust governance frameworks, regulatory oversight akin to an FAA model for AI in healthcare, and the importance of setting clear visions and timelines for AI adoption. The emphasis should be on ensuring safety, consistency, and the ethical use of AI in healthcare, alongside fostering innovation and maintaining patient empowerment.
Breakout 4 – AI for Learning and Teaching – key takeaways
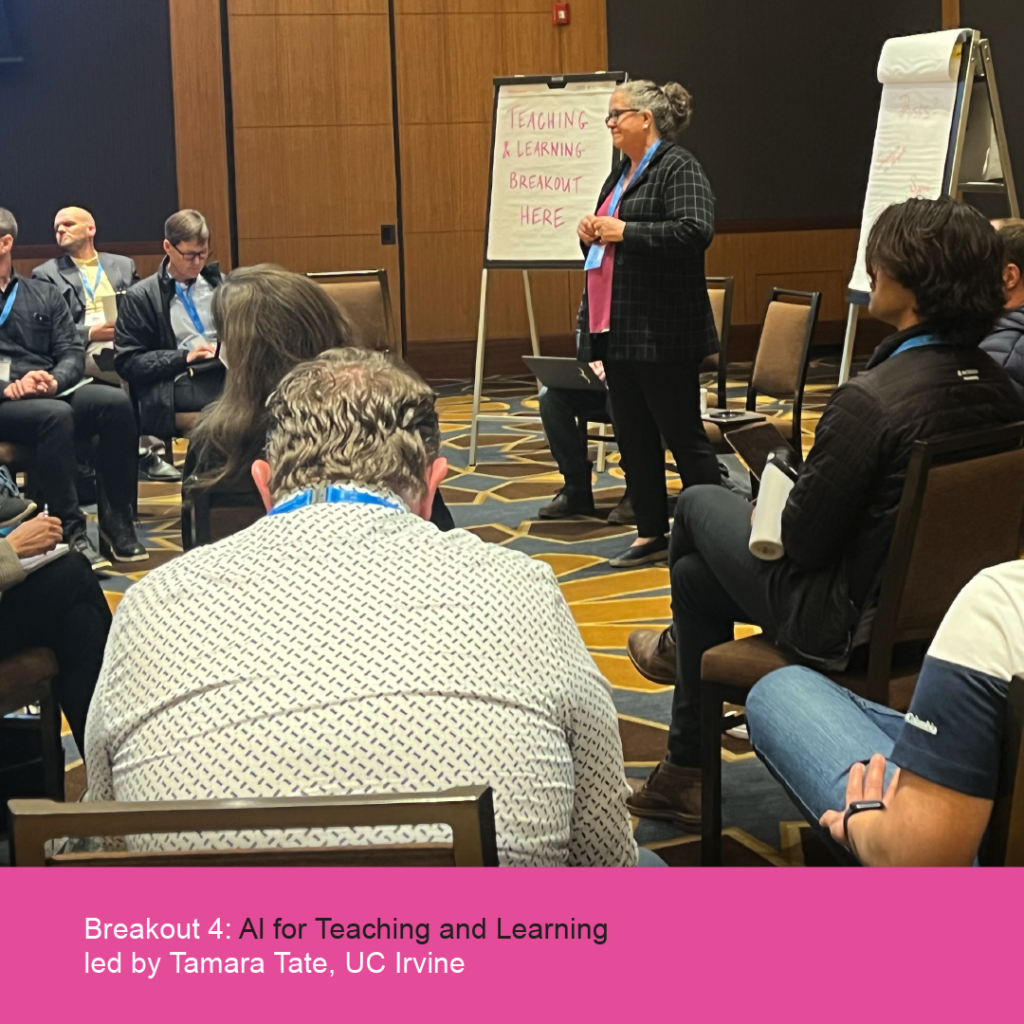
- Significant Playground/Sandbox Space in classrooms. There are numerous examples of faculty thoughtfully applying LLM/Generative AI in the curriculum, both as a way to learn the mechanisms of the tools while also promoting critical conversation.
- Make sure you are between the AI and your students. Harnessing the AI requires significant scaffolding to be successful, or it can be detrimental. Make students consider: is the AI better than all human options available at that time and place? Help students use AI with purpose, but not reliance.
- Scale the thoughtfulness from #1 across the system. We have been using AI for many years: admissions, EdTech vendors, early alert…and there has not been clarity around equity of access or protocols for use from the system. Leading with compassion and concern is critical.
Breakout 5 – AI for the Creative Sector – key takeaways
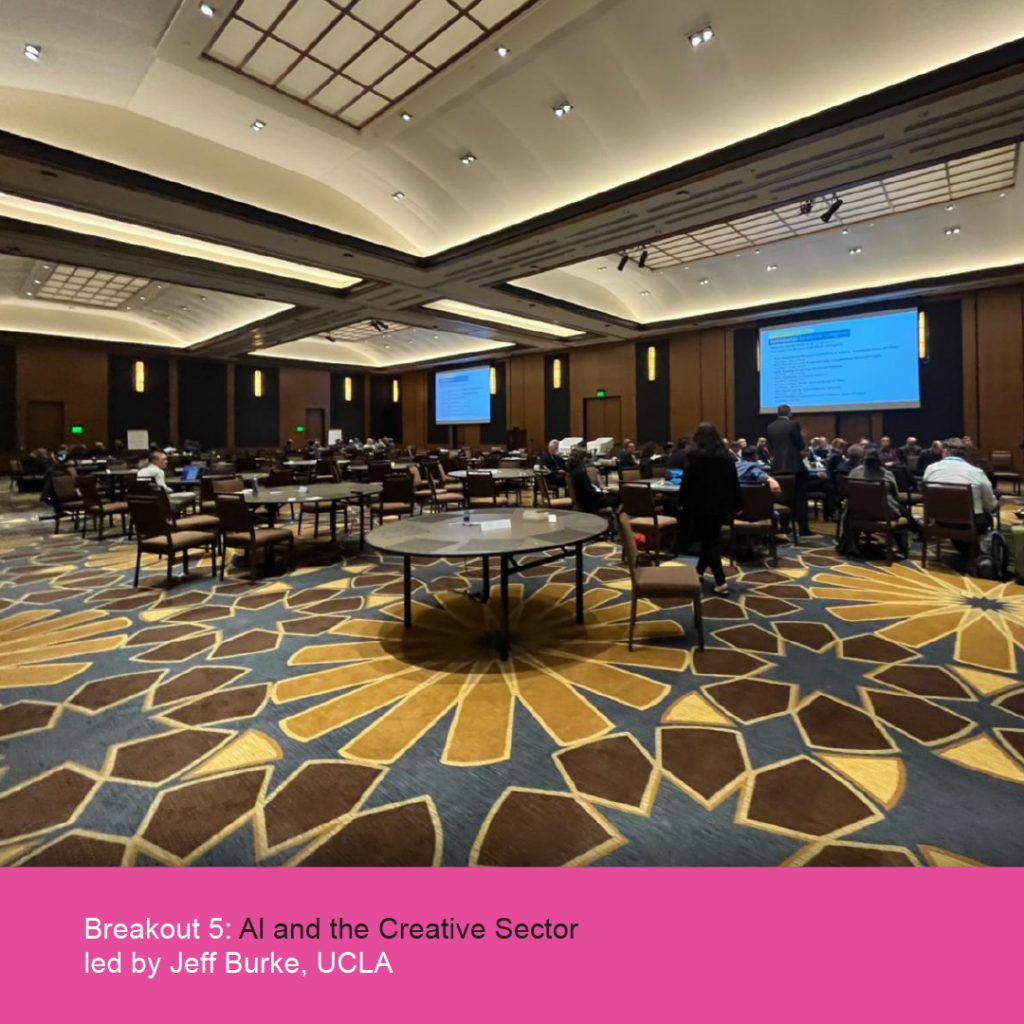
- Highlight a systemwide push for AI literacy that has a hands-on component.
- Make infrastructure available for faculty and students to think about AI as new creative material that they can participate in designing.
- Encourage porosity across disciplines, particularly between STEM and arts/humanities, including funding that enables graduate students from different disciplines to come together.
Breakout 6 – AI in Computational Research Applications and Research Integrity – key takeaways
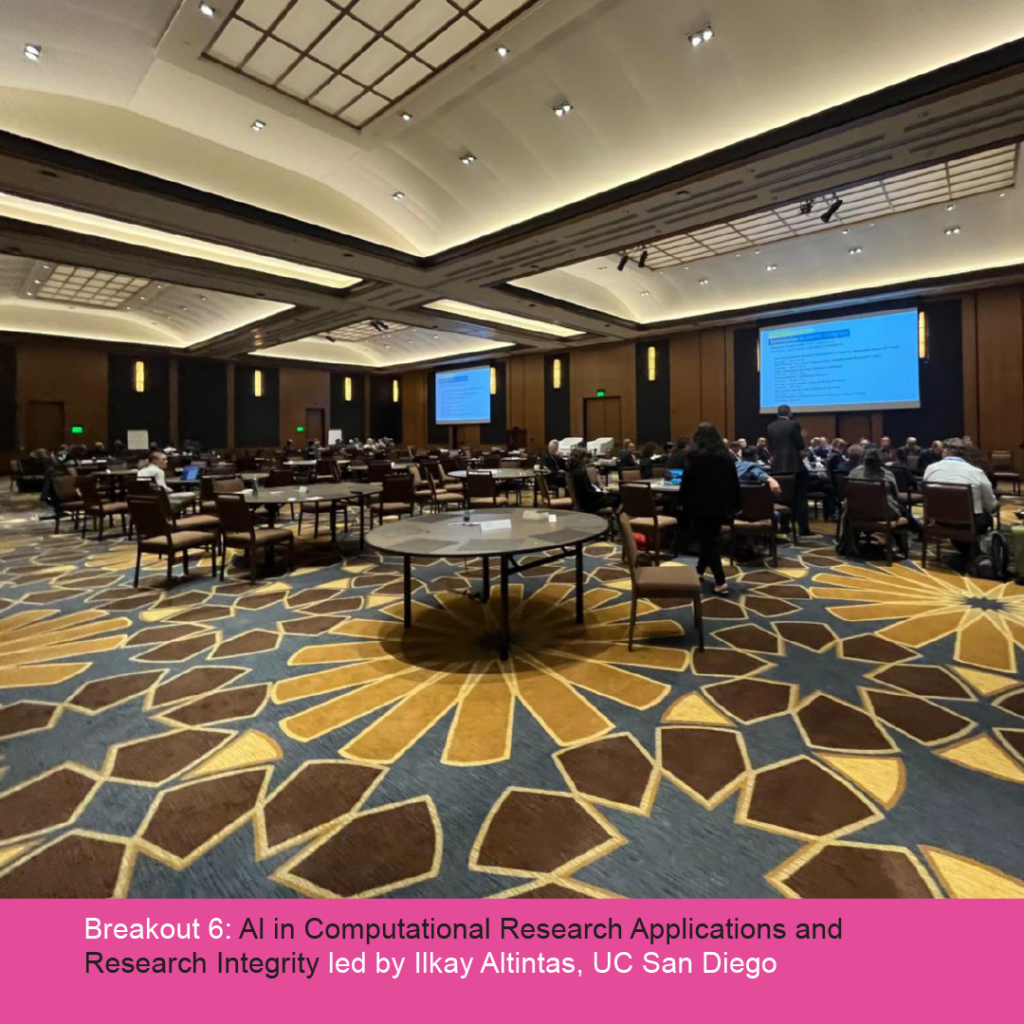
- AI in research spans all aspects of the scientific process, from research proposals to data collection and analysis to publication of results. AI can possibly support research infrastructure and be a research subject itself (e.g., cognitive impact of AI on youth).
- AI has implications on research integrity, both as something that needs to be validated and then also a potential tool to examine for research falsification. In addition, IRBs are not equipped to consider AI implications on human subjects.
- AI infrastructure can be costly – how do we consider the costs for data storage, including opportunities to collect information today that may not be imminently useful but possibly useful for future research. In addition, how do we address pending restrictions from publishers that may limit access for articles which would limit the benefit or increase the costs of using AI.
Breakout 7 – Ethical Review and Human Subjects Research Involving AI – key takeaways
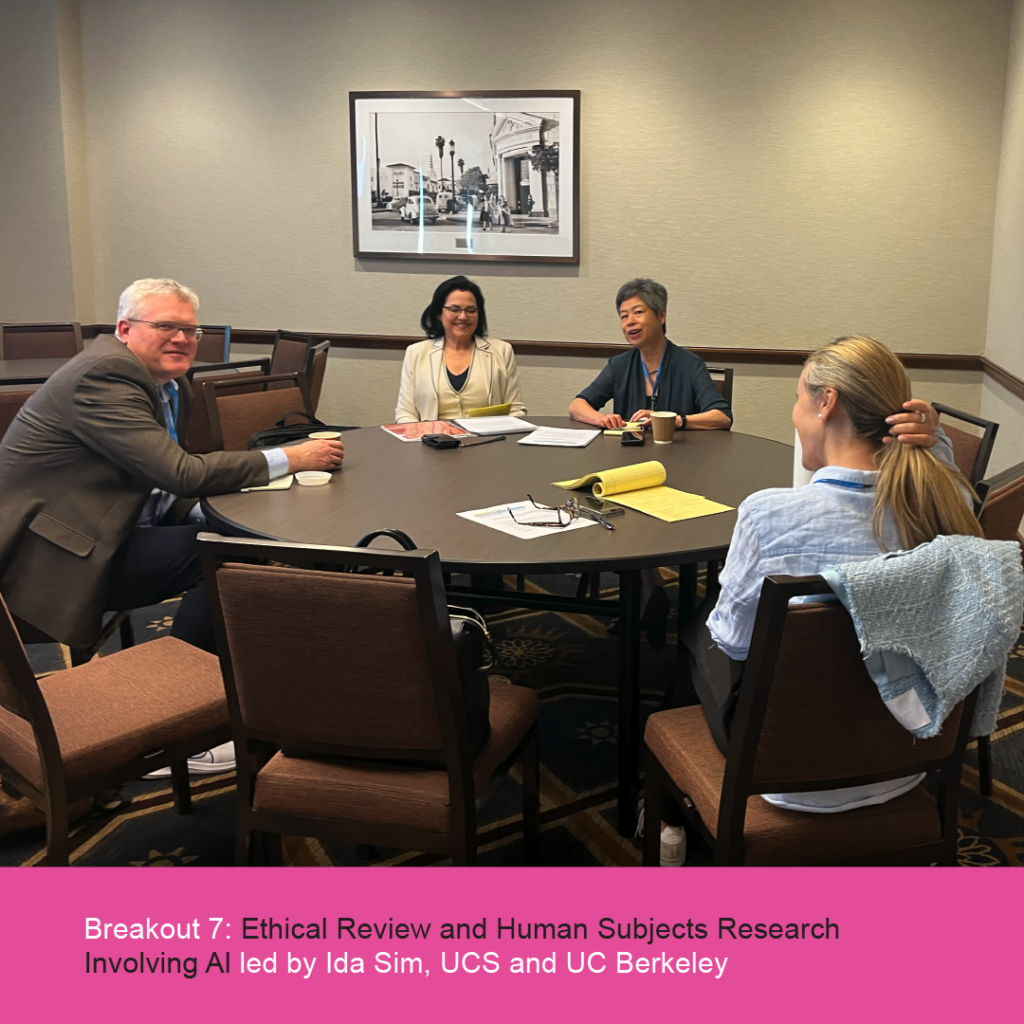
- The existing Institutional Review Board (IRB) process needs to have clearer policies and guard rails including a risk-based framework that could leverage work that’s ongoing mostly by the Presidential Working Group on Al. IRBs will need more resources and tools in anticipation of more studies involving Al that need additional review, including faculty expertise, staff support and new expertise that has yet to be developed so that IRBs can more effectively ensure ethics of human subjects research involving Al.
- The use of Al is experimental, humans (e.g., staff, students, patients) are subjects in that process, and there are risks that are not subject to IRB review. This presents risks and the UC should think about how we can manage these risks.
- Research involving Al also presents risks at the community and societal level. We should consider convening a process to assess and mitigate community and societal-level risks.